As an example of the WEIGHT statement, suppose 20 people
are asked to estimate the size of an object 30 cm wide. Each person
is placed at a different distance from the object. As the distance
from the object increases, the estimates should become less precise.
The SAS data set SIZE
contains the estimate (ObjectSize) in centimeters at each distance
(Distance) in meters and the precision (Precision) for each estimate.
Notice that the largest deviation (an overestimate by 20 cm) came
at the greatest distance (7.5 meters from the object). As a measure
of precision, 1/Distance, gives more weight to estimates that were
made closer to the object and less weight to estimates that were made
at greater distances.
The following statements
create the data set SIZE:
options nodate pageno=1 linesize=64 pagesize=60;
data size;
input Distance ObjectSize @@;
Precision=1/distance;
datalines;
1.5 30 1.5 20 1.5 30 1.5 25
3 43 3 33 3 25 3 30
4.5 25 4.5 36 4.5 48 4.5 33
6 43 6 36 6 23 6 48
7.5 30 7.5 25 7.5 50 7.5 38
;
The following PROC MEANS
step computes the average estimate of the object size while ignoring
the weights. Without a WEIGHT variable, PROC MEANS uses the default
weight of 1 for every observation. Thus, the estimates of object size
at all distances are given equal weight. The average estimate of
the object size exceeds the actual size by 3.55 cm.
proc means data=size maxdec=3 n mean var stddev;
var objectsize;
title1 'Unweighted Analysis of the SIZE Data Set';
run;
The next two PROC MEANS
steps use the precision measure (Precision) in the WEIGHT statement
and show the effect of using different values of the VARDEF= option.
The first PROC step creates an output data set that contains the variance
and standard deviation. If you reduce the weighting of the estimates
that are made at greater distances, the weighted average estimate
of the object size is closer to the actual size.
proc means data=size maxdec=3 n mean var stddev;
weight precision;
var objectsize;
output out=wtstats var=Est_SigmaSq std=Est_Sigma;
title1 'Weighted Analysis Using Default VARDEF=DF';
run;
proc means data=size maxdec=3 n mean var std
vardef=weight;
weight precision;
var objectsize;
title1 'Weighted Analysis Using VARDEF=WEIGHT';
run;
The variance of the
ith
observation is assumed to be

and

is the weight for the
ith
observation. In the first PROC MEANS step, the computed variance is
an estimate of

. In the second PROC MEANS step, the computed variance
is an estimate of
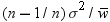
, where

is the average weight. For large n, this value is
an approximate estimate of the variance of an observation with average
weight.
The following statements
create and print a data set with the weighted variance and weighted
standard deviation of each observation. The DATA step combines the
output data set that contains the variance and the standard deviation
from the weighted analysis with the original data set. The variance
of each observation is computed by dividing Est_SigmaSq (the estimate
of

from the weighted analysis when VARDEF=DF) by each
observation's weight (Precision). The standard deviation of each observation
is computed by dividing Est_Sigma (the estimate of

from the weighted analysis when VARDEF=DF) by the
square root of each observation's weight (Precision).
data wtsize(drop=_freq_ _type_);
set size;
if _n_=1 then set wtstats;
Est_VarObs=est_sigmasq/precision;
Est_StdObs=est_sigma/sqrt(precision);
proc print data=wtsize noobs;
title 'Weighted Statistics';
by distance;
format est_varobs est_stdobs
est_sigmasq est_sigma precision 6.3;
run;